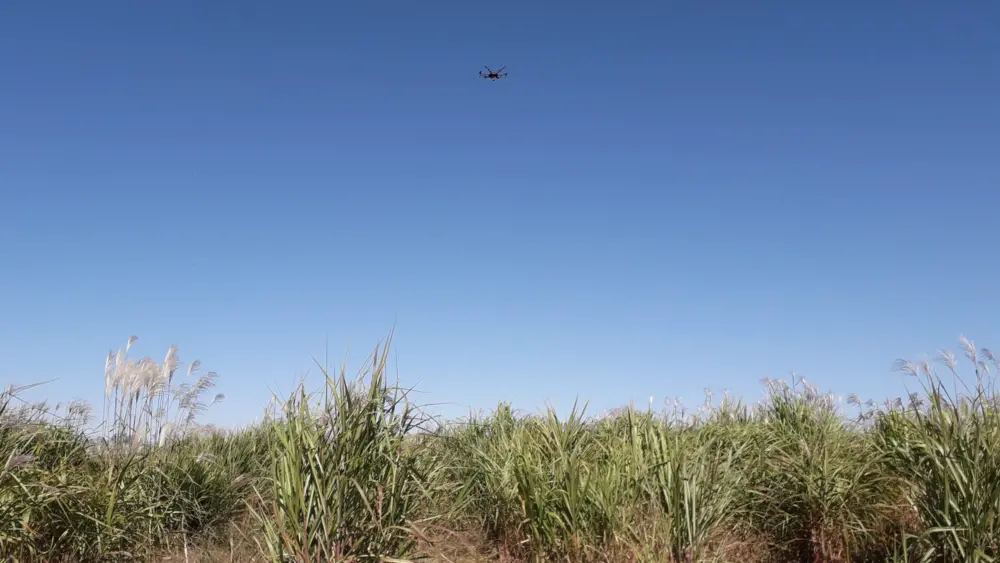
However, breeding must become faster and more efficient if it is to reach the potential for sustainable and resilient biomass production in miscanthus. A key bottleneck in the process is the ability to measure the growth of thousands of varieties of the crop in the field and select the small number of varieties that perform best. This requires new, sophisticated technologies for capturing data and analyzing it. A study by researchers at the Center for Advanced Bioenergy and Bioproducts Innovation (CABBI) demonstrated how unmanned aerial vehicles (UAVs, or drones) combined with cutting-edge machine learning methods can assist the selection of the best candidate genotypes in miscanthus breeding programs. The team used neural nets — computer systems modeled on the human brain and nervous system — to analyze very high-resolution aerial imagery and identify key miscanthus traits during the crop’s growing season. In particular, the CABBI researchers highlighted that using neural networks that are designed to analyze data in three-dimensions (two dimensions in space, plus time) allowed better estimates of crop traits (flowering time, height, and biomass production) than traditional neural networks that analyze data in only two dimensions in space. This allowed them to leverage information on how each of the thousands of plants in the field change over time. In addition, the three-dimensional neural network proved able to automatically perform aspects of the image analysis process (i.e., finding plants in the image) which in many other cases requires substantial manual intervention that would slow the process down.